To sign up for our daily email newsletter, CLICK HERE
Table Of Contents:
I. Inception of Math Solver AI
- Technological Advancements
III. Applications of Math Solver AI
- Impact on Education
- Challenges and Future Directions
- Tools and Platforms
In the realm of artificial intelligence (AI), one area that has seen remarkable progress is the development of math solver AI systems. These intelligent assistants are designed to tackle mathematical problems, ranging from simple arithmetic to complex calculus, with speed and accuracy. This article delves into the evolution of math solver AI, exploring its inception, technological advancements, and the impact it has had on education and beyond.
I. Inception of Math Solver AI
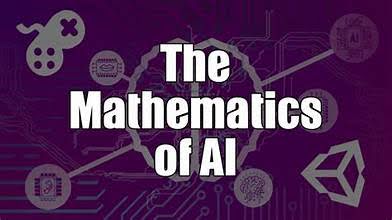
Early Attempts in Math Solver AI:
The early attempts at creating math solver AI can be traced back to the early stages of computing when researchers began exploring the potential of computers to automate mathematical calculations. These initial endeavours focused on developing rudimentary algorithms capable of solving online computer class and mathematical problems. Although these early systems were limited in scope and functionality, they laid the foundation for subsequent developments in the field of math solver AI.
During this period, programmers experimented with simple algorithms to perform arithmetic operations such as addition, subtraction, multiplication, and division. These algorithms were often implemented in low-level programming languages and executed on early computing devices, which had limited computational power and memory capacity.
Despite their simplicity, these early math solver AI systems represented an important step forward in the automation of mathematical tasks. They demonstrated the feasibility of using computers to perform routine calculations and laid the groundwork for more sophisticated approaches to mathematical problem-solving.
Emergence of Symbolic Computation:
A significant milestone in the evolution of math solver AI was the emergence of symbolic computation. Symbolic computation involves manipulating mathematical expressions symbolically rather than numerically. Unlike numerical methods, which operate on numerical values, symbolic computation operates on symbols, variables, and mathematical operations.
The development of symbolic computation algorithms enabled AI systems to perform complex mathematical operations with precision and efficiency. These algorithms could manipulate algebraic expressions, solve equations symbolically, and perform symbolic integration and differentiation.
Symbolic computation revolutionized the field of math solver AI by enabling computers to handle abstract mathematical concepts and perform sophisticated mathematical reasoning. It provided a powerful framework for developing advanced math solver AI systems capable of tackling a wide range of mathematical problems.
Integration of Machine Learning:
As the field of AI advanced, researchers began integrating machine learning techniques into math solver AI systems. Machine learning algorithms enable these systems to learn from data, adapt to new challenges, and improve their performance over time.
One approach to integrating machine learning into math solver AI involves training algorithms on large datasets of mathematical problems and solutions. These algorithms learn patterns and relationships in the data, allowing them to generalize to new problems and make accurate predictions.
Another approach is to use machine learning algorithms to enhance the problem-solving capabilities of math solver AI systems. These algorithms can analyze the structure of mathematical problems, identify relevant features, and generate effective solution strategies.
The integration of machine learning marked a significant leap forward in the capabilities of math solver AI. It enabled these systems to handle more complex and varied mathematical problems, adapt to changing contexts, and provide more accurate and efficient solutions.
In conclusion, the evolution of math solver AI has been characterized by early attempts at creating rudimentary algorithms, the emergence of symbolic computation, and the integration of machine learning techniques. These developments have paved the way for increasingly sophisticated math solver AI systems capable of tackling a wide range of mathematical problems with precision and efficiency.
II. Technological Advancements
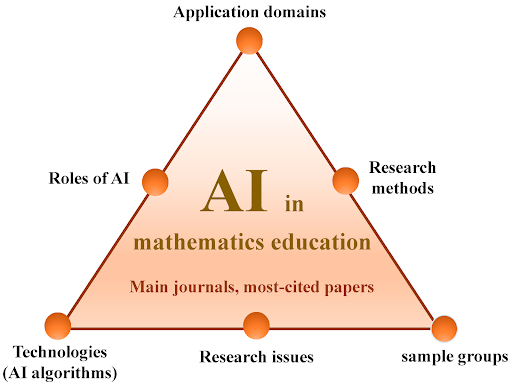
Natural Language Processing (NLP):
Natural Language Processing (NLP) is a key technological advancement that has greatly enhanced math solver AI systems. NLP allows users to input mathematical problems in plain language, enabling a more intuitive and accessible interaction with the AI system. Traditionally, users needed to input mathematical expressions in symbolic notation, which could be daunting for those unfamiliar with mathematical syntax. NLP bridges this gap by allowing users to describe problems in everyday language.
The integration of NLP into math solver AI systems involves several components. First, the system must be capable of understanding and parsing natural language input. This requires sophisticated algorithms for text processing, including tokenization, part-of-speech tagging, and syntactic analysis. Once the input is parsed, the system must identify the mathematical components and relationships within the text. This involves semantic analysis to extract the mathematical meaning from the natural language description.
Once the mathematical problem is understood, the math solver AI system can apply appropriate algorithms to solve it. This may involve symbolic computation, numerical methods, or a combination of both, depending on the nature of the problem. The system then generates a solution in a format that is easy for the user to understand, often including step-by-step explanations to aid comprehension.
NLP has significantly expanded the usability of math solver AI systems, making them accessible to a wider audience. Users no longer need to be proficient in mathematical notation to benefit from these tools. Instead, they can simply describe their problems in everyday language and receive accurate solutions in return.
Computer Vision:
Computer vision technology has also played a crucial role in advancing math solver AI systems. By enabling AI systems to interpret handwritten equations, graphs, and diagrams, computer vision expands the range of input formats that can be processed by math solver AI.
Handwritten mathematical expressions pose a unique challenge for traditional input methods. Unlike typed text, handwritten characters can vary widely in size, shape, and orientation, making them difficult to interpret accurately. Computer vision algorithms address this challenge by analyzing the visual features of handwritten input and converting it into a digital format that can be processed by the math solver AI system.
The incorporation of computer vision into math solver AI systems involves several stages. First, the system must detect and locate handwritten text within the input image. This may involve techniques such as edge detection, contour analysis, and optical character recognition (OCR). Once the text is detected, the system must segment it into individual characters and symbols, identify their meanings, and reconstruct the mathematical expression.
Computer vision technology enables users to input mathematical problems in various formats, including handwritten notes, scanned documents, or even whiteboard sketches. This flexibility makes math solver AI systems more versatile and adaptable to different use cases. It also enhances the user experience by allowing for more natural and intuitive interactions with the AI system.
Algorithmic Enhancements:
Continuous refinement of algorithms is another crucial aspect of advancing math solver AI technology. As mathematical problems become increasingly complex, the algorithms used to solve them must also evolve to meet the growing demand for speed, accuracy, and efficiency.
Algorithmic enhancements in math solver AI systems can take many forms. Researchers may develop new algorithms that improve the performance of existing methods or explore novel approaches to mathematical problem-solving. This may involve optimizing algorithms for specific types of problems, reducing computational complexity, or leveraging parallel processing techniques to improve scalability.
Additionally, algorithmic enhancements may focus on improving the user experience by reducing latency, providing more informative feedback, or optimizing the presentation of results. For example, algorithms may be developed to generate step-by-step explanations of the solution process, helping users understand the underlying concepts and techniques.
Overall, algorithmic enhancements play a crucial role in advancing math solver AI technology, enabling these systems to tackle increasingly complex problems with greater speed and accuracy. By continuously refining and optimizing algorithms, researchers can ensure that math solver AI systems remain at the forefront of mathematical problem-solving capabilities.
III. Applications of Math Solver AI
Education Sector:
Math solver AI has brought about a revolution in the education sector, transforming the way students learn and engage with mathematical concepts. These AI systems serve as invaluable tools that provide instant feedback and personalized assistance to students, aiding them in improving their problem-solving skills and understanding of mathematical concepts.
One of the primary benefits of math solver AI in education is its ability to offer instant feedback. Unlike traditional methods where students must wait for teachers to grade their assignments or exams, math solver AI systems can provide immediate feedback on students’ work. This instant feedback helps students identify and correct mistakes more efficiently, leading to faster learning and mastery of mathematical concepts.
Moreover, math solver AI systems offer personalized assistance tailored to each student’s individual learning needs and pace. These systems can adapt to students’ strengths, weaknesses, and learning styles, providing targeted support and guidance. For example, if a student is struggling with a particular concept, the AI system can offer additional explanations, examples, or practice problems to reinforce understanding.
Math solver AI has become an integral part of mathematics education, supplementing traditional teaching methods and enhancing the learning experience for students. By providing instant feedback, personalized assistance, and a wealth of resources, these AI systems empower students to take control of their learning and achieve academic success in mathematics.
Professional Fields:
In addition to its impact on education, math solver AI also plays a crucial role in various professional fields. Engineers, scientists, economists, and other professionals rely on math solver AI to streamline calculations, analyze data, and solve complex mathematical problems.
One of the key benefits of math solver AI in professional fields is its ability to save time and effort. These AI systems can perform mathematical calculations and analyses much faster than humans, enabling professionals to focus on higher-level tasks and innovation in their respective fields. For example, engineers can use math solver AI to quickly design and optimize complex systems, while scientists can use it to analyze experimental data and simulate complex phenomena.
Moreover, math solver AI systems provide a level of accuracy and consistency that is difficult to achieve manually. By automating mathematical tasks, these AI systems reduce the risk of human error and ensure reliable results. This is particularly important in fields where precision and accuracy are paramount, such as engineering, finance, and scientific research.
Overall, math solver AI has become an indispensable tool for professionals across various fields, enabling them to work more efficiently, make informed decisions, and drive innovation. By leveraging the power of AI technology, professionals can tackle complex mathematical problems with confidence and achieve their goals more effectively.
Research and Development:
Math solver AI also plays a crucial role in research and development across diverse fields such as physics, chemistry, and economics. Researchers rely on math solver AI to analyze data, simulate experiments, and solve complex mathematical problems, enabling them to gain insights into complex phenomena, explore new theories, and advance scientific knowledge.
One of the key advantages of math solver AI in research and development is its ability to handle large volumes of data and complex mathematical models. These AI systems can process and analyze massive datasets much faster than traditional methods, allowing researchers to uncover patterns, trends, and correlations that may not be apparent otherwise.
Moreover, math solver AI enables researchers to simulate complex experiments and scenarios, providing valuable insights into the behavior of systems and phenomena. For example, physicists can use math solver AI to simulate the behavior of particles in high-energy collisions, while chemists can use it to model chemical reactions and predict molecular structures.
Overall, math solver AI has become an indispensable tool for researchers in their quest to push the boundaries of knowledge and innovation. By providing powerful computational capabilities and advanced mathematical tools, these AI systems empower researchers to tackle the most challenging problems and make breakthrough discoveries across a wide range of fields.
IV. Impact on Education
Accessibility:
One of the most significant impacts of math solver AI on education is its role in promoting accessibility. Traditional methods of teaching mathematics often present barriers to students with diverse learning needs and abilities, including those with disabilities or learning difficulties. Math solver AI systems help break down these barriers by providing support and assistance tailored to individual students’ needs.
Students with disabilities, such as visual impairments or dyslexia, may face challenges when it comes to reading and interpreting mathematical notation. Math solver AI systems address these challenges by offering alternative input methods, such as voice commands or tactile interfaces, that cater to different learning styles and preferences. For example, students with visual impairments can use speech-to-text technology to input mathematical problems verbally, while students with dyslexia can benefit from simplified explanations and visual aids provided by the AI system.
Furthermore, math solver AI systems enable students to access personalized assistance and resources that cater to their specific needs and abilities. These AI systems can adapt their output to accommodate different learning styles, preferences, and levels of proficiency, ensuring that all students have equal access to educational opportunities. By leveling the playing field, math solver AI empowers students to succeed in mathematics education and reach their full potential.
Personalized Learning:
Math solver AI facilitates personalized learning by adapting to individual learning styles, preferences, and pacing. Traditional classroom settings often follow a one-size-fits-all approach to instruction, where all students are expected to progress at the same pace and master the same concepts within a predefined timeframe. However, this approach fails to account for the diverse needs and abilities of students, leading to gaps in understanding and disengagement.
Math solver AI systems address this challenge by providing customized feedback and guidance tailored to each student’s unique learning profile. These AI systems can adapt their teaching strategies based on factors such as students’ performance, preferences, and areas of difficulty, providing targeted support and scaffolding when needed. For example, a struggling student may receive additional practice problems and explanations to reinforce understanding, while an advanced student may be presented with more challenging tasks to stimulate intellectual curiosity.
By allowing students to learn at their own pace and according to their individual needs, math solver AI fosters greater engagement, motivation, and academic achievement. Students feel empowered and supported in their learning journey, leading to improved confidence and self-efficacy in mathematics education.
Empowering Educators:
Math solver AI also empowers educators by automating routine tasks and providing valuable insights into students’ learning progress and challenges. Traditional teaching methods often involve time-consuming activities such as grading assignments, providing feedback to students, and tracking individual progress manually. Math solver AI systems streamline these processes by automating routine tasks, freeing up valuable time and resources for educators to focus on higher-level tasks such as lesson planning, curriculum development, and individualized instruction.
Furthermore, math solver AI systems provide educators with valuable data and analytics that can inform instructional decision-making. These AI systems track students’ performance, identify areas of difficulty, and offer actionable insights into effective teaching strategies and interventions. Educators can use this information to tailor instruction to meet the diverse needs of their students, differentiate instruction based on individual learning profiles, and provide targeted support to students who need it most.
Overall, math solver AI empowers educators to create more inclusive and effective learning environments, where all students have equal opportunities to succeed in mathematics education. By automating routine tasks, providing personalized feedback and guidance, and offering valuable insights into student learning, math solver AI systems support educators in their mission to inspire and empower the next generation of mathematicians, scientists, and innovators.
V. Challenges and Future Directions
Algorithmic Bias:
Algorithmic bias is a critical challenge facing math solver AI systems. These systems may inadvertently perpetuate or exacerbate existing biases present in the data used to train them. Bias can manifest in various forms, such as racial, gender, socioeconomic, or cultural bias, and can lead to unfair or discriminatory outcomes.
The problem of algorithmic bias arises when AI systems learn patterns from biased data and reproduce those biases in their decision-making processes. For example, if historical data used to train a math solver AI system disproportionately represents certain demographic groups or contains inherent biases, the system may learn to favor those groups or perpetuate stereotypes.
Addressing algorithmic bias in math solver AI systems is essential to ensure fairness, equity, and inclusivity in education and beyond. This requires careful attention to the data used to train AI models, as well as rigorous testing and evaluation to identify and mitigate biases in the algorithms.
Techniques for mitigating algorithmic bias include:
1. Diverse and Representative Data:
Ensuring that training data is diverse and representative of the population it seeks to serve can help mitigate bias. This may involve collecting data from a wide range of sources and ensuring that it includes diverse perspectives and experiences.
2. Bias Detection and Mitigation:
Employing techniques such as fairness-aware machine learning algorithms can help detect and mitigate biases in AI systems. These techniques involve analyzing the output of the AI system to identify biases and adjusting the model’s parameters to reduce or eliminate them.
3. Transparency and Accountability:
Promoting transparency and accountability in the development and deployment of math solver AI systems can help identify and address bias. This may involve documenting the decision-making processes, disclosing the sources of data used to train the system, and providing mechanisms for users to report bias or discrimination.
By addressing algorithmic bias in math solver AI systems, we can ensure that these systems promote fairness, equity, and inclusivity in education and contribute to positive social outcomes.
Ethical Considerations:
Ethical considerations surrounding the use of math solver AI systems pose significant challenges that must be addressed to ensure responsible deployment and usage. These considerations encompass a range of issues, including academic integrity, privacy, and data security.
One ethical concern is academic integrity, particularly in educational settings. Math solver AI systems have the potential to facilitate cheating and academic dishonesty if not used responsibly. Students may be tempted to rely on AI systems to solve homework assignments or exams without understanding the underlying concepts, undermining the educational process.
Privacy is another ethical consideration when it comes to math solver AI systems. These systems may collect and process sensitive personal data, such as students’ mathematical performance or learning preferences. Protecting the privacy of users and ensuring that data is handled responsibly and in accordance with relevant privacy laws and regulations is essential.
Data security is also a significant ethical concern, particularly given the potential for malicious actors to exploit vulnerabilities in math solver AI systems. Protecting against data breaches, unauthorized access, and other security threats requires robust cybersecurity measures and protocols.
Ensuring that math solver AI systems adhere to ethical principles and guidelines is essential to build trust and confidence among users. This may involve implementing transparent and accountable decision-making processes, respecting user privacy and data rights, and promoting responsible usage practices.
By addressing ethical considerations surrounding the use of math solver AI systems, we can foster a culture of responsible AI deployment and usage that maximizes the benefits while minimizing the risks.
Advancements in Quantum Computing:
The future of math solver AI holds promise for further innovation and advancement, particularly with emerging technologies such as quantum computing. Quantum computing has the potential to revolutionize math solver AI by solving previously intractable problems at unparalleled speeds.
Unlike classical computers, which use binary bits to represent information as either 0 or 1, quantum computers use quantum bits or qubits, which can exist in multiple states simultaneously. This enables quantum computers to perform calculations at exponentially faster speeds than classical computers, particularly for problems involving large datasets or complex mathematical operations.
Quantum computing could enable math solver AI systems to tackle even more complex mathematical problems and unlock new possibilities in mathematics and beyond. For example, quantum algorithms could be developed to solve optimization problems, factor large numbers, or simulate quantum systems with unprecedented accuracy and efficiency.
Furthermore, quantum computing could enhance the capabilities of math solver AI systems in fields such as cryptography, finance, and scientific research. By leveraging the power of quantum computation, these AI systems could provide insights and solutions to problems that are currently beyond the reach of classical computing.
However, quantum computing also presents challenges and limitations that must be addressed. These include technical hurdles such as error correction, noise reduction, and scalability, as well as ethical considerations surrounding the potential societal impacts of quantum technologies.
Overall, advancements in quantum computing hold tremendous potential for the future of math solver AI, enabling these systems to push the boundaries of mathematical problem-solving and unlock new opportunities for innovation and discovery. By embracing quantum computing technologies, we can accelerate progress in AI and mathematics and address some of the most pressing challenges facing society.
VI. Tools and Platforms
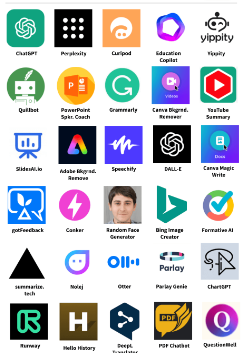
Wolfram|Alpha:
Wolfram|Alpha is a computational knowledge engine developed by Wolfram Research. Unlike traditional search engines that index web pages, Wolfram|Alpha computes answers to queries by synthesizing data from various sources and applying sophisticated algorithms. It is renowned for its vast knowledge base and its ability to provide answers to a wide range of queries, including mathematical problems.
Math-solving Capabilities:
One of the prominent features of Wolfram|Alpha is its math-solving capabilities. Users can input mathematical problems in natural language or symbolic form, and Wolfram|Alpha will compute and provide solutions, step-by-step explanations, visualizations, and interactive graphs. Whether it’s solving algebraic equations, calculus problems, statistics, or complex mathematical concepts, Wolfram|Alpha can tackle a wide array of mathematical queries.
Other Functionalities:
In addition to math-solving, Wolfram|Alpha offers a plethora of other functionalities. It can provide answers and insights related to science, technology, engineering, finance, linguistics, and more. Users can inquire about historical events, geographical information, nutritional data, and even receive real-time data on weather, stocks, and demographics. Wolfram|Alpha also powers various applications and services, including Wolfram Language, Wolfram|Alpha Notebook Edition, and Wolfram|Alpha Pro.
Photomath:
Photomath is a popular mobile app designed to help users solve mathematical problems using their smartphone camera. Leveraging computer vision technology, Photomath can interpret handwritten equations captured through the device’s camera and provide step-by-step solutions and explanations in real-time.
How Photomath Works:
Users simply point their smartphone camera at a handwritten or printed math problem, and Photomath’s computer vision algorithms analyze the image to recognize and understand the mathematical expression. Once the equation is identified, Photomath proceeds to solve it step-by-step, guiding users through the solution process with explanations and annotations. Users can interact with the solution, exploring each step in detail and gaining insights into the problem-solving methodology.
Key Features:
– Step-by-step solutions: Photomath provides detailed explanations for each step of the solution process, helping users understand the underlying concepts and techniques.
– Real-time feedback: Users receive immediate feedback as they interact with the solution, enabling them to identify errors and learn from their mistakes.
– Interactive learning: Photomath offers a dynamic learning experience, allowing users to explore different problem-solving strategies and concepts at their own pace.
– Multiple language support: Photomath supports multiple languages, making it accessible to users worldwide.
Symbolab:
Symbolab is an online math solver that specializes in symbolic computation, graphing, and equation-solving. It offers a comprehensive set of tools and features designed to assist students, educators, and professionals in solving mathematical problems and visualizing mathematical concepts.
Symbolic Computation:
Symbolab excels in symbolic computation, which involves manipulating mathematical expressions symbolically rather than numerically. This approach enables Symbolab to handle complex algebraic expressions, equations, and inequalities, providing exact solutions and simplifications.
Graphing and Visualization:
Symbolab allows users to graph functions, equations, and inequalities, visualizing mathematical concepts in a graphical format. Users can customize the appearance of graphs, adjust parameters, and explore the behavior of functions interactively.
Equation-solving Features:
Symbolab offers a variety of equation-solving features, including solving linear and nonlinear equations, systems of equations, differential equations, and more. Users can input equations in natural language or symbolic form, and Symbolab will compute solutions and provide step-by-step explanations.
Educational Resources:
Symbolab provides educational resources and tutorials to help users learn and master mathematical concepts. These resources include practice problems, examples, and explanations covering a wide range of topics in algebra, calculus, trigonometry, and other mathematical disciplines.
Microsoft Math Solver:
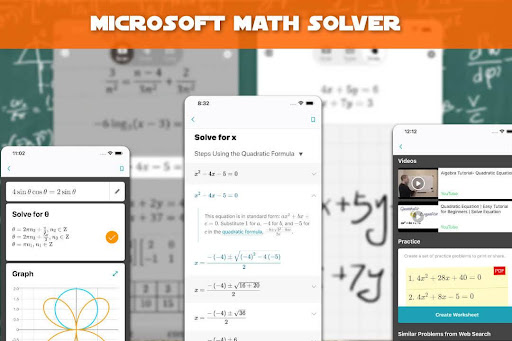
Microsoft Math Solver is an AI-powered tool integrated within Microsoft Office applications, including Word, OneNote, and Teams. It provides math assistance to users, allowing them to input mathematical problems in various formats and receive step-by-step solutions, graph plotting, and interactive learning resources.
Integration with Microsoft Office:
Microsoft Math Solver seamlessly integrates with Microsoft Office applications, making it easily accessible to users as they work on documents, notes, or presentations. Users can input mathematical problems directly into their Office documents and receive real-time assistance and feedback from Microsoft Math Solver.
AI-powered Solutions:
Microsoft Math Solver leverages artificial intelligence algorithms to analyze mathematical problems and generate step-by-step solutions. These solutions are presented in a clear and concise manner, guiding users through the problem-solving process and providing insights into the underlying concepts.
Interactive Learning Resources:
In addition to step-by-step solutions, Microsoft Math Solver offers interactive learning resources such as graph plotting, practice problems, and educational videos. These resources enable users to explore mathematical concepts further, reinforce their understanding, and improve their problem-solving skills.
Multi-format Input:
Microsoft Math Solver supports input in various formats, including text, handwritten notes, or images. Users can input mathematical problems using a keyboard, stylus, or camera, making it convenient and flexible to use across different devices and platforms.
In conclusion, Wolfram|Alpha, Photomath, Symbolab, and Microsoft Math Solver are powerful tools that leverage AI and computational techniques to assist users in solving mathematical problems, visualizing mathematical concepts, and enhancing learning experiences. Whether it’s performing symbolic computation, analyzing handwritten equations, graphing functions, or integrating with productivity software, these math solver tools offer valuable resources and support to students, educators, and professionals alike.
Author Bio:
Umer Rashid is a dynamic AI researcher specializing in mathematics, with a keen focus on developing innovative solutions to complex problems. Holding a . in Computer Science, Umer’s expertise lies in the intersection of mathematics and artificial intelligence. He is passionate about leveraging AI technology to enhance education and promote accessibility. Umer is committed to addressing ethical considerations and algorithmic bias in AI systems, ensuring they serve the greater good. With a dedication to excellence and a vision for a brighter future, Umer continues to push the boundaries of possibility in AI research. Additionally, Umer is known for his contributions to online education platforms, including Take my online calss for me, where he seeks to revolutionize the learning experience through AI-driven solutions.